Mastering Mean Reversion Algo Trading: A Guide to Quantitative Strategies and Algorithms
This comprehensive guide to mean reversion algo trading explores how to use algorithmic and quantitative strategies to capture predictable price reversions. Covering the difference between algo and quant trading, key components of mean reversion setups, and essential tools like moving averages and Bollinger Bands, this post provides actionable insights for traders looking to automate reversion trades. With step-by-step tips on coding, backtesting, and optimizing a mean reversion trading algorithm, this guide is perfect for traders eager to leverage data-driven strategies for consistent returns.
5 min read
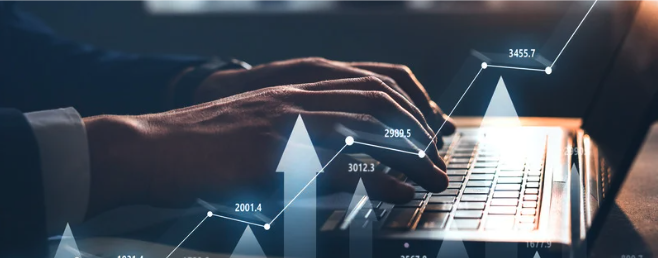
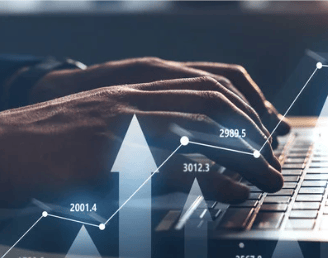
The Complete Guide to Mean Reversion Algo Trading: Strategies, Tools, and Tips for Success
Mean reversion algo trading is a strategy designed to capitalize on the tendency of asset prices to return to a historical average or “mean” over time. This approach, also known as mean reverting algorithmic trading or mean reversion quantitative trading, is popular in various markets, from stocks and forex to commodities. By using data-driven methods, mean reversion trading algorithms help traders automate their strategies, identifying and acting on deviations from the mean. In this guide, we’ll explore the fundamentals of algorithmic trading mean reversion strategies and how you can leverage them for a systematic trading edge.
Algo vs. Quant – What’s the Difference?
When it comes to mean reversion trading, understanding the difference between algorithmic (algo) trading and quantitative (quant) trading is key to building effective strategies:
- Algo Trading: Algo trading refers to using computer programs to automate trades based on a set of predefined rules. In mean reversion algo trading, the algorithm executes trades automatically once specific conditions are met, such as when the price deviates significantly from the mean.
- Quant Trading: Quantitative (quant) trading focuses on using mathematical models and historical data to develop trading strategies. A mean reversion quant strategy is grounded in data analysis to identify reversion patterns and the statistical likelihood of prices moving back toward the mean.
In practice, quant trading often informs the research and rules used in algo trading, while algo trading automates these rules. Together, these approaches create a data-driven, systematic edge in mean reversion trading.
Understanding Mean Reversion in Algorithmic Trading
What Is Mean Reversion?
Mean reversion is the idea that prices will fluctuate around a long-term average or "mean" level. In algorithmic trading mean reversion strategies, traders capitalize on price deviations, assuming that any overextended move away from the mean will eventually reverse, returning the price to its average. This statistical tendency is attractive for traders because it provides predictable trade setups based on historical patterns.
Why Use Mean Reversion in Algorithmic Trading?
By using a mean reversion trading algorithm, traders can automate these reversion setups, entering and exiting trades without manual input. This approach allows traders to act on even the smallest price deviations quickly, making it ideal for high-frequency or intraday trading setups. By leveraging data and quantifiable thresholds, mean reverting algorithmic trading strategies can systematically exploit price movements without emotional bias.
Key Components of a Mean Reversion Quant Strategy
1. Defining the Mean and Setting Thresholds
To define the “mean,” a mean reversion quant strategy often uses moving averages or regression lines to determine where prices should theoretically revert. Thresholds—typically set as standard deviations or percentage bands around the mean—indicate how far the price must deviate before a reversion trade is triggered. Bollinger Bands or Keltner Channels, which create dynamic boundaries around the mean, are popular tools for identifying these extremes.
2. Entry and Exit Rules in Mean Reversion Algorithms
For effective mean reversion quantitative trading, clear entry and exit rules are essential:
- Entry: Enter when the price deviates a certain distance from the mean, signaling an overbought or oversold condition.
- Exit: Take profit once the price returns to the mean or reaches a predefined level.
These rules help ensure trades are only triggered when price extremes occur, filtering out noise and increasing the likelihood of successful reversion trades.
3. Risk Management and Position Sizing
Risk management is key to mean reverting algorithmic trading. This includes setting stop losses just beyond the extreme deviation to limit potential losses and adjusting position sizes based on volatility. Dynamic adjustments, often calculated using the ATR (Average True Range), allow the algorithm to adapt to changing market conditions without overexposing the portfolio.
Tools and Indicators for Mean Reversion Quantitative Trading
1. Moving Averages and Statistical Indicators
Moving averages (SMA, EMA) serve as a baseline for calculating the mean in mean reversion trading algorithms. Statistical tools like Bollinger Bands or Keltner Channels provide dynamic upper and lower boundaries, which are crucial for determining when a price is overextended from the mean and setting up reversion trades.
2. Volume and Momentum Indicators
Indicators like the RSI (Relative Strength Index) or MACD (Moving Average Convergence Divergence) validate overbought or oversold conditions. By confirming mean reversion setups with these indicators, traders reduce the risk of false signals and improve entry accuracy.
3. Automation Platforms and Coding Considerations
To implement a mean reversion trading algorithm, platforms like Python, TradingView (Pine Script), or QuantConnect offer tools for developing and backtesting strategies. Backtesting on historical data helps verify the strategy’s reliability, allowing traders to refine entry/exit rules, thresholds, and risk management parameters before live trading.
Developing and Optimizing a Mean Reversion Algo Trading Strategy
1. Coding a Mean Reversion Trading Algorithm
Building a mean reversion algo trading strategy typically involves coding the following steps:
- Data Processing: Import and clean historical price data, calculating moving averages, ATR, or other chosen indicators.
- Signal Generation: Define entry and exit rules based on price deviations from the mean, using conditions for confirmation.
- Execution Logic: Set up a mechanism to place trades, apply stop-losses, and monitor real-time data.
2. Backtesting and Optimizing Parameters
Before going live, backtest the algorithmic trading mean reversion strategy on historical data across different timeframes and market conditions. Walk-forward optimization—where the strategy is tested on one segment of data and then validated on another—helps prevent overfitting. Track key metrics like Sharpe ratio, win rate, and drawdown to gauge effectiveness.
3. Live Deployment and Monitoring
Once the algorithm is optimized, deploy it in a live environment, closely monitoring performance and making adjustments as needed. A mean reversion algo trading strategy requires ongoing assessment, especially in volatile markets, to ensure parameters remain effective and aligned with market conditions.
Pros and Cons of Mean Reversion Algo Trading
Advantages
- Consistent Profits in Range-Bound Markets: Mean reversion algorithms excel in stable, sideways markets, where prices repeatedly revert to the mean.
- Automation Reduces Emotional Trading: By automating decisions, traders eliminate emotional biases that can lead to poor judgment.
- Customization for Risk Management: Parameters can be adjusted for position sizing, stop-loss levels, and trade frequency, allowing traders to tailor the strategy to their risk tolerance.
Risks and Limitations
- Vulnerability in Trending Markets: When markets strongly trend in one direction, mean reversion strategies can struggle, as prices may not revert as expected.
- Risk of Overfitting: Optimizing the algorithm too closely to historical data can lead to overfitting, making the strategy less effective in real-world trading.
- Regular Monitoring and Adjustments Needed: Even automated strategies require periodic adjustments to adapt to changing market conditions and maintain profitability.
As You Can See
Mean reverting algorithmic trading offers a powerful, systematic approach for traders seeking consistent gains in range-bound markets. By defining clear parameters, setting thresholds, and automating trades, mean reversion quant strategies provide a data-driven edge. To maximize success, traders should combine careful backtesting, risk management, and ongoing monitoring.
Ready to Add $50 or More to Your Daily Income?
If you’re serious about mastering reversion to mean trading, visit our About RTM page, where you'll find essential education, information, tips, and tools to perfect your strategy. Don’t miss out—dive into more in-depth content and sharpen your skills ASAP!
Extreme to Mean
Low Risk, High Reward - Reversion Done Right
Join Our Newsletter 📧
© 2024. All rights reserved.
Support@ExtremetoMean.com
Unlock exclusive trading tips, tools, and strategies—sign up for our newsletter and take the first step toward consistent profits!
All content is for educational purposes only and not financial advice. Trading carries risk, including potential loss of capital.